Personalized, Precise and Data-Driven: Precision Medical Education is Here
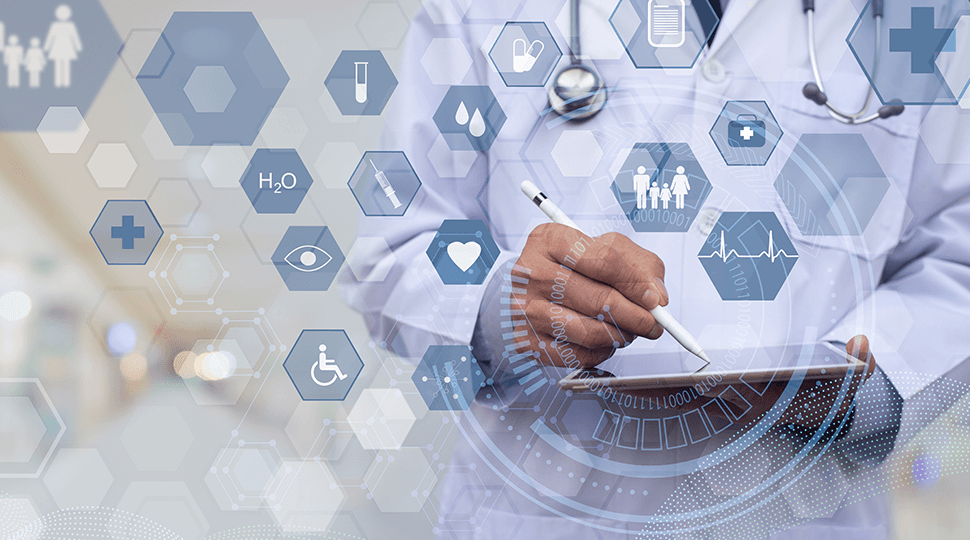
June 6, 2023
Note: this blog post was originally published in 2020 on One45’s blog, and has been updated to include the latest insights on Precision Medical Education.
When medical education tries to widen access to its learners, it becomes clear that using the same teaching methods for everyone has its limitations. Uniform instruction does not take into account each individual’s complex learning goals or needs. But Precision Medical Education does.
Movement from novice to master in medicine is a journey that is unique to every healthcare practitioner. In an era of rapid change and focus on widening pathways into medicine, medical schools must be prepared to accommodate different learning needs, speeds, and techniques. The interventions and remediation steps appropriate for one learner are not necessarily drive best results with another. Interventions need to be appropriately tailored to produce benefit for individual students.
Luckily, the foundations for personalized, precise medical education are in place. Thanks to the digitization and organization of medical education data, we now have the foundation we need to use data science and get real-time insights about everything from admissions to residencies. In addition, advancements in educational research combined with these new data insights guides us towards a new era in medical education.
Education is following new norms established elsewhere in healthcare
Medical education is following in the footsteps of other established healthcare fields by utilizing the combination of big data and human knowledge to advance the practice. In healthcare, initiatives aimed at personalizing an individual’s patient care are called “Precision Medicine.” As opposed to a one-size-fits-all approach, Precision Medicine takes into account individual variability in genes, environment, and lifestyle for each person — leading to disease treatment and prevention strategies that consider the differences between individuals.
Other fields like pharmacogenetics and multiomics are also combining data, data science, and specialist expertise to personalize treatment as a means to improve efficacy.
Education, enabled by technology, is also speeding towards mass personalization. As a reflection of the precision medicine work being done to evolve and advance the practice of healthcare, we’ve chosen to use the term Precision Medical Education for the data-driven insights and practices that are emerging across healthcare education. A working definition of Precision Medical Education, or PME, is:
An ethical, science-based approach to optimizing and individualizing the learning environment, training, and career outcomes of medical professionals based on multiple data points, data science, and research.
Just as the patient must be at the center of personalized (precision) medicine, the learner must be at the heart of precision medical education. To varying degrees, PME will also impact medical schools, institutions, healthcare providers, industry associations, and other stakeholders.
What Precision Medical Education aims to achieve
Individualized learning
PME seeks to provide a personalized experience to each trainee based on that individual’s particular attributes and preferences, as reflected in their data and by their actions. This will provide insights into creating an ideal learning environment.
Optimized career outcomes
PME aims to positively influence the choices that confront medical trainees in medical school, residency, and beyond. There are obvious and non-obvious links between an individual’s choices and their future resiliency, likelihood of burnout, job satisfaction, and earning potential. PME seeks to tease out these links and provide clear insights with a decision-support system to aid those individuals in making choices that set them up for long-term success.
Efficacy and efficiency in training variables
Training can be rated in terms of time, quality, and cost. The goal of PME is to decrease the cost or resources of training, and reduce the time of training (if possible) while increasing quality.
In order to achieve these goals and outcomes, PME at its very foundation MUST be:
Ethical
A foundational element of a precision service is the assumption that vast quantities of individuals’ data will be used by algorithms to make individualized predictions. This is certainly the case with PME, but this data-centric view of individuals is rife with problems. Ethics, and an adaptive view of ethics when it comes to algorithmic bias, is an essential and foundational requirement for the development and proliferation of PME.
Evidence-based
Medical education is built on education research. In some ways, the development of PME algorithms is like the “translational research” of medical education. Data science can translate educational research into practice in novel ways. As such, the duty to be open to criticism, to collaborate and test your work, and to live by a scientific code is critical when developing and practicing PME.
Beyond “just data”
Today, PME and other precision initiatives rely mostly on data and data science to provide personalized insights. This is the core technical change that makes PME possible today. At the same time, a heavy reliance on “just data” is too narrow. A working definition of PME should leave the door open to other, non-data-centric aspects of the learner experience — whatever they may be and whenever they arise.
Evolving to a more PME-Centric approach
In this 2020 article, you’ll discover details into the key phases medical schools go through as they transition to a more PME-centric approach:
- A breaking down of data silos and the surfacing of insights from integrated data to inform decision making
- Establishment of process and policies around data management and governance
- The evolution of internal talent and knowledge from data literacy (ability to analyze a set of data and draw useful conclusions about what happened) to data fluency (ability to turn those conclusions into testable hypotheses about what will happen and enable the CQI process)
Why PME is developing now
The foundation for Precision Medical Education includes:
- Appropriate data
- People with sufficient knowledge of medical education and data science that can responsibly bring positive and effective change
Key developments in both of these areas have moved PME from a desirable future state to something that can be achieved now.
Medical education data is primed for algorithmic use
Medical education has a long history with standards: accreditation standards, standard competency sets, work standards, and curriculum standards. Medical education data tied to standards has largely been captured digitally into internal and external data instruments over the last 20 years. Due to the accreditation process, it’s mostly available for on-demand reporting.
Machine learning algorithms need structured data sets to train on in order to generate intelligent insights. Medical education has been building comprehensive, structured digital datasets for the past 20 years. As more medical schools are compelled to make better use of their data, their operational datasets will become increasingly suitable for advanced analytics and precisely modeled recommendations.
Research and researchers are emerging that will inform Precision Medical Education
To do precision health, you need health data (mostly genomics) and physicians working alongside data scientists. Instead of health data, PME requires medical education data. Instead of physicians working with data scientists, PME requires medical education researchers and practitioners working with data scientists to support learners and their programs.
As medical education research goes, so too do the technology-infused tools and approaches to problems in the MedEd industry. Just like the research of Olle ten Cate and others on entrustable professional activities (EPAs) is leading to a wide scale reorientation towards more competency-based medical education (CBME), medical education data science research will lead to an embrace of the educational methods required for PME.
A few examples of recent papers highlight a growing trend:
A call to investigate the relationship between education and health outcomes using big data
Published in 2018, this paper (and three related papers) call for more investigation into the link between education and health outcomes using advanced analytics. The papers highlight the growing volumes of healthcare data that can be tied back to individual clinicians.
Signatures of medical student applicants and academic success
Published in 2020, this paper uses machine learning approaches to cluster students into distinct groups with unique characteristics. From the abstract (emphasis mine): “The big data approach presented here can more sensitively uncover success potential since it takes into account the inherent heterogeneity within the student population.”
Schools are starting to recognize the importance and opportunity of PME-related work. They are increasingly looking at their data as an important, structured asset. They are collaborating with (or training) people with advanced data capabilities.
Until now, this PME approach has been limited to a small number of schools with enough resources to try and manage the data and who were fortunate enough to have researchers to dive in to find the needles in the haystack.
But today, we’re at a moment where the people and the data sets necessary to achieve something approaching PME are coming together across medical education. Products like Analytics by Acuity Insights are enabling schools to tackle PME-related projects without the previous need for a large-scale team of technologists and data scientists.
Over the last year, Acuity (who acquired One45) customers have:
- used Analytics to revise long-held grading policies to fit with the clearer picture integrated data provides
- repeatedly chosen to open up previously closed data silos to more and more varied stakeholders — creating the foundations of a data democracy within some medical schools.
- begun using longitudinal data sets for machine-learning around student cohort segmentation
- kicked off conversations and research around data benchmarking and sharing
The opportunity ahead
As these developments continue, medical schools will collectively be in a position to start tackling some of the biggest questions in medical education — with data. Questions like:
- How do I ensure 100 percent matching of my learners into best-aligned residency programs?
- How do I select the applicants that will stay in my community and choose to serve our mission?
- How do I identify struggling students and create a custom, personalized set of interventions to help them?
- Which aspects of my program are truly adding value for learners and our broader mission?
Enabling Precision Medical Education is at the core of our mission at Acuity. As we continue to build upon our solutions, we won’t be just providing data to programs but contextualizing that data so programs and learners know what to take action on. We won’t be aiming to increase the cost of medical school for learners but helping schools understand where to truly deploy their resources — and where they’re not necessary. We won’t be trying to hide our research and algorithms, rather we will be building a large-scale research community that is fair, transparent, and evidence-based.
Tomorrow’s healthcare professionals will require resilience, empathy, communication, collaboration, and digital aptitude. As educators, we must remember that our students are not like weeds, capable of growth in any soil. Most require the right conditions to become their best selves, and that is what PME will enable for aspiring professionals.
Related Articles
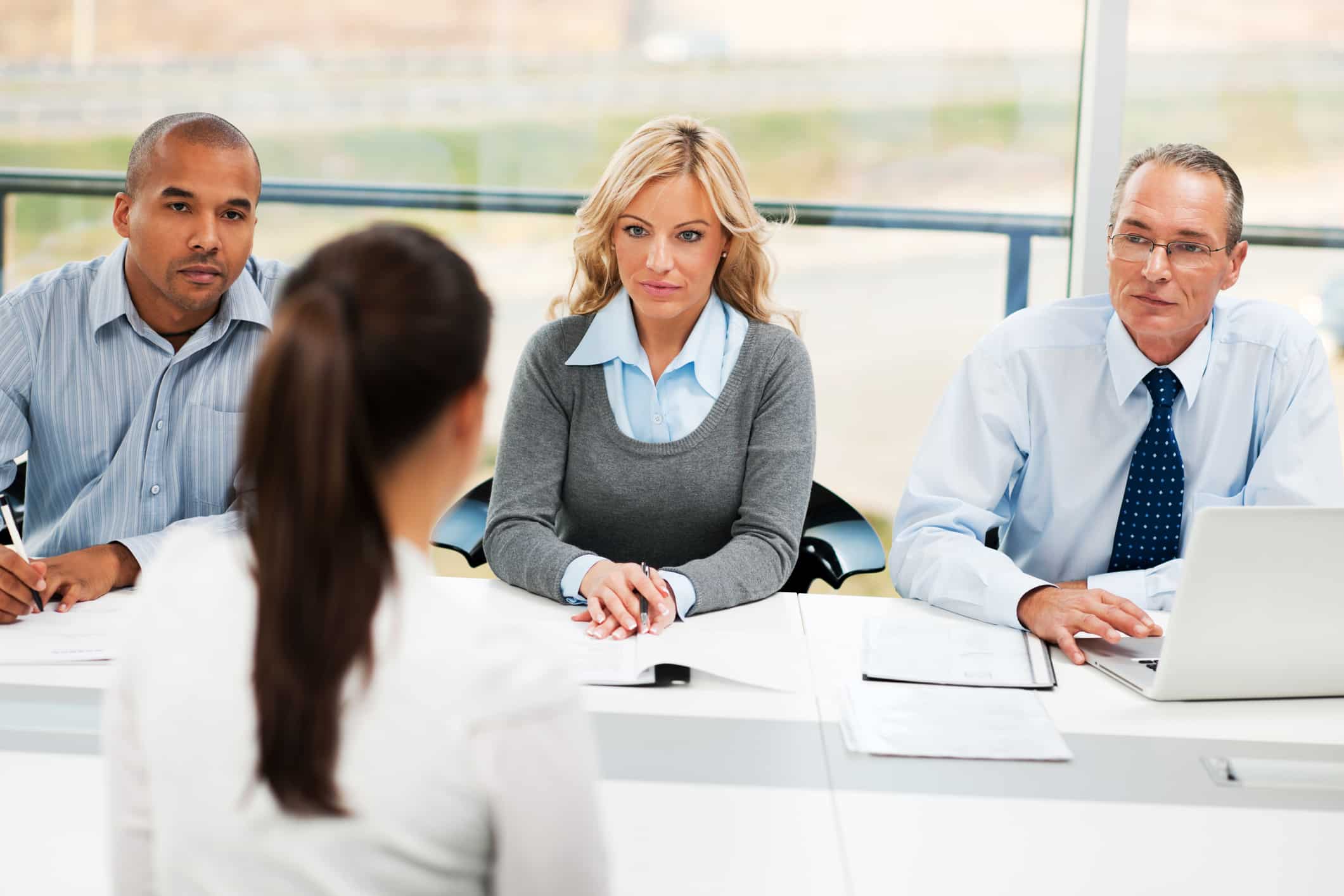
How interviews could be misleading your admissions...
Most schools consider the interview an important portion of their admissions process, hence a considerable…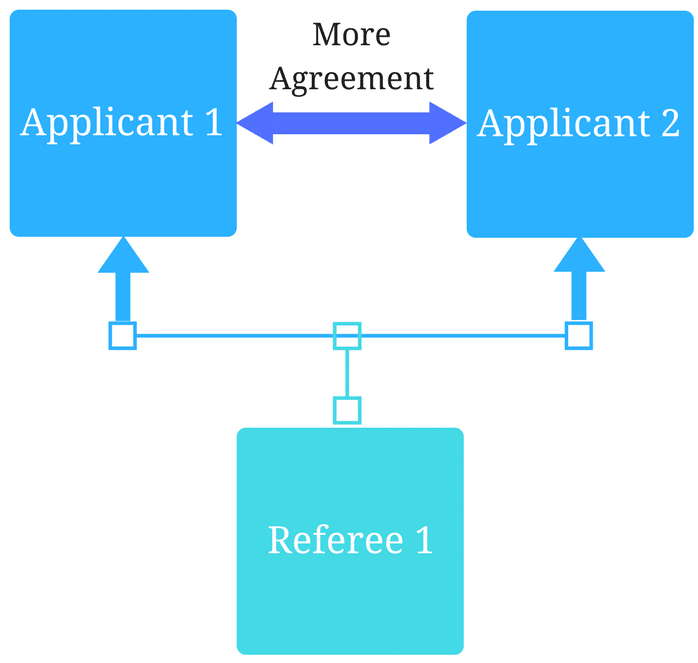
Reference letters in academic admissions: useful o...
Because of the lack of innovation, there are often few opportunities to examine current legacy…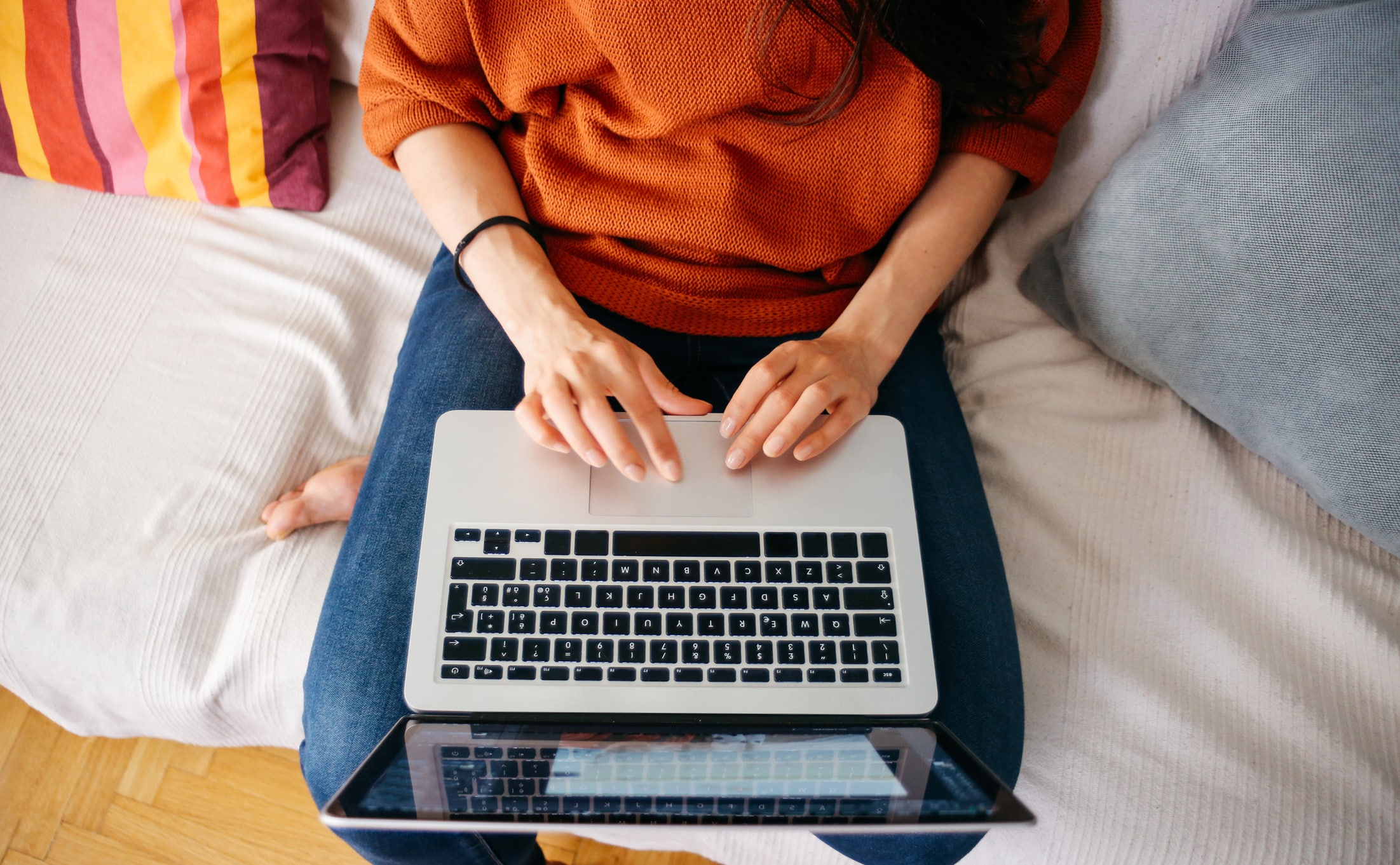