Make (and keep) your MedEd data healthy – a treatment plan
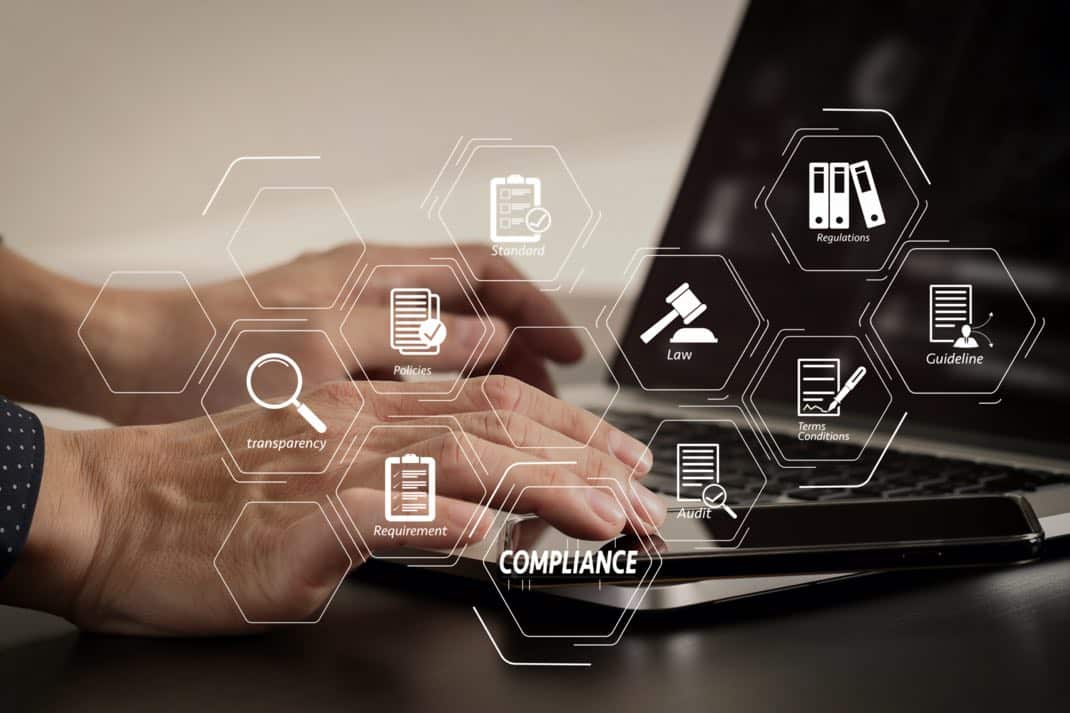
April 1, 2021
Previously in how healthy is your school’s data?, we discussed the signs to look for when assessing the health of your MedEd institution’s data. In short, to make the best use of your data, it must maintain high levels of consistency, accuracy, completeness, and conformance. What’s key here is that this process is always ongoing. As new data is added to the system every day, processes and checks must be put in place to ensure they meet these four aspects of quality. Without ongoing routine maintenance, your data’s quality will slowly deteriorate (i.e., data entropy) to the point of being unusable. Good data quality is attained through strong data governance that creates a framework of standards, processes, and metrics by which your data is managed.
Data governance
All efforts around data quality must first start with strong data governance – the people, standards, metrics, and processes responsible for keeping data healthy. It is critical that governance has broad support across your institution, most importantly at the leadership level. Leadership will need to agree to giving the governance committee the ability to assign data stewardship and data ownership roles to existing employees throughout the institution. Without leadership’s buy-in, efforts to harmonize your institution’s data from across many disparate systems will likely prove futile.
With support from leadership, a data governance committee made up of representatives from across departments can begin to meet to discuss standards, processes, metrics, and also assign roles of data stewardship to key individuals. An initial audit of what standards and processes are in place can help to assess what’s already going well and what needs improvement in your data quality strategy. Meeting regularly will ensure standards and processes are monitored and improved upon, while keeping data quality top of mind for every member.
Standards
Standards are the rules your institution decides to apply to your data. These are unique to your school and your use cases for the data, but oftentimes revolve around ensuring consistent naming and identifiers are used across systems. Standards might look something like, “Each student is represented by their institution’s alphanumeric identifier and not their email address. All ‘student id’ or ‘student number’ fields in our systems must use this identifier for all students.” Standards should also include:
- definitions of the data that the standard addresses. If we use the previous example, the standards would detail what the student ID is, how it’s used, and its importance in the systems it’s represented within.
- provisions for what to do in edge cases. In the previous example, for instance, what happens for visiting students who aren’t assigned an institutional student ID? Is there an alternative ID that is acceptable in this case? Missing these edge cases can create confusion among administrators of data and lead to bad data quality.
- which system should be the source of truth for the data? Using the same example, if student A has one student ID in one system and another different student ID in another system, which one is correct?
Once standards have been vetted and agreed upon by the data governance committee, they should live within a standards guidebook that can be disseminated across data administrators in the institution.
Metrics
Once standards have been created, measures of how well these standards are adhered to can be used to quantify how your data quality process is performing. Since perfection is unattainable, setting realistic goals on these measures allows your institution to define how important conformance is for each individual standard. This can be done by benchmarking newly-minted standards early on to determine where your institution is and where it needs to get to. One example metric might measure how many students are found in X systems where they are expected to have entries. A goal of, for example, 90 percent presence across all systems could then be used to determine whether action be taken or not to rectify data quality issues in missing or mismatched students across systems.
Processes
Processes to maintain data quality fall into two camps: proactive and reactive processes. Proactive processes are oftentimes automated by setting up or programming business rules in the different systems in use at the institution to prevent data from entering that doesn’t meet the defined quality standards. This is the ideal type of process, but is often difficult or impossible to implement due to the need for third-party systems and IT departments to support these rules. An example of a proactive process might be setting up a rule in your LMS that ensures the email entered for a student is their institution-issued email address, thus preventing unexpected email addresses from ever being added to the system.
Most of the time, reactive processes will be necessary to enforce standards. While these can be automated, they are more likely to be manual checks and audits of your systems to determine standards compliance, followed by a request from data stewards to fix offending data points. For example, an institution may have multiple different identifiers assigned to a student. To ensure all systems use the same student identifier convention, data stewards audit each system on a regular basis, looking for student identifiers that don’t match a certain format. If non-conforming identifiers are found, they request these identifiers be fixed by administrators of the offending systems. Have clear processes in place is important to prevent data quality issues becoming a “hot potato” that gets passed around and not fixed.
Conclusion
Data quality is often given second-billing to the data collection itself. But, make no mistake; strong data governance will help ensure the efforts put into data collection are not wasted. Without concerted efforts to monitor data quality and maintain conventions and standards, schools will often struggle to unlock the rich value out of the data they have. This is increasingly important as schools look to unify this data within centralized data warehouses such as Acuity Analytics, where data quality issues become glaringly apparent. While it may look like a challenge to nurse data back to health, it’s likely much more challenging dealing with the consequences of taking no action at all, such as data misinterpretations, conflicting data accounts, and missed opportunity costs of using the data to its full potential.
Related Articles
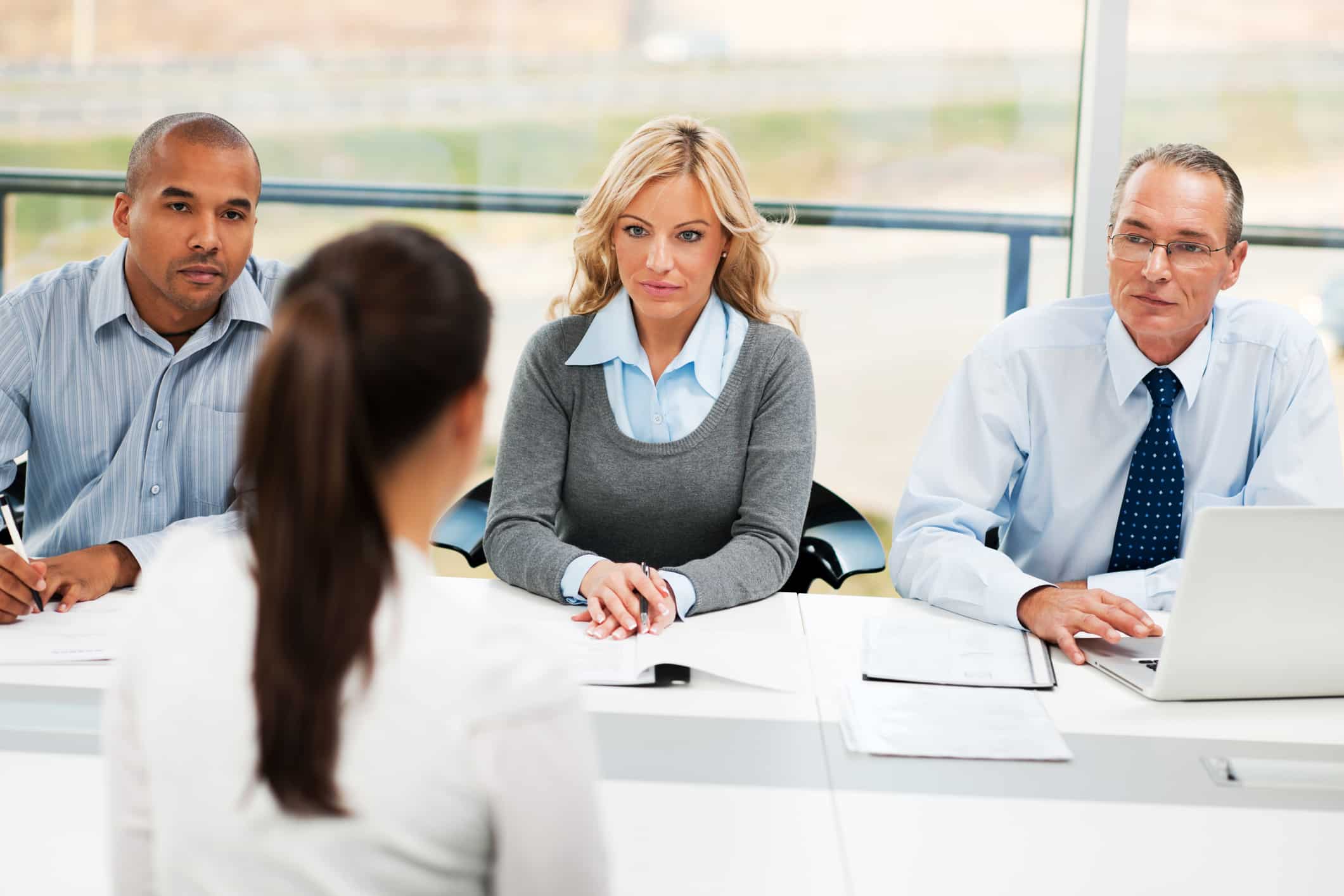
How interviews could be misleading your admissions...
Most schools consider the interview an important portion of their admissions process, hence a considerable…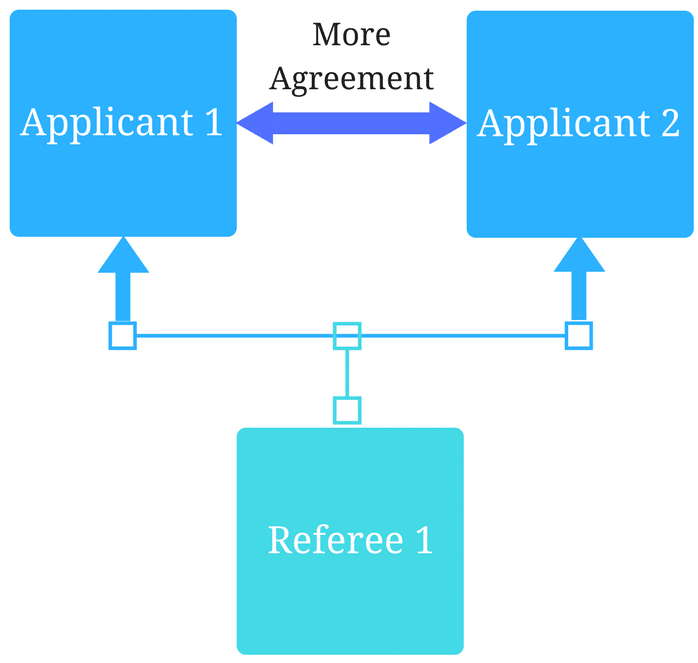
Reference letters in academic admissions: useful o...
Because of the lack of innovation, there are often few opportunities to examine current legacy…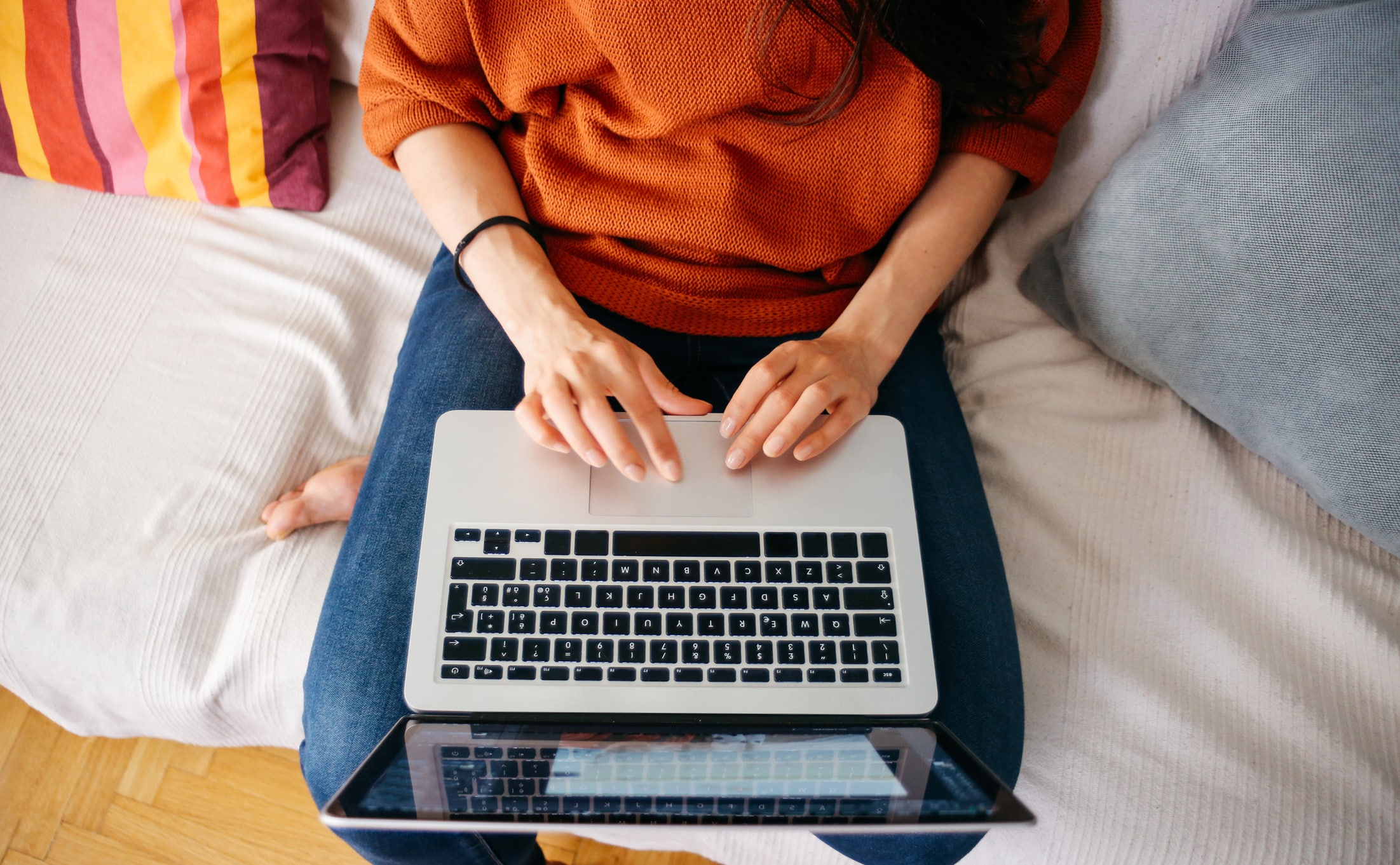